- The Deep View
- Posts
- ⚙️ A description, prediction and prescription: AI as a normal technology
⚙️ A description, prediction and prescription: AI as a normal technology

Good morning. Whether you were finishing up Passover or celebrating Easter, hope you all had a holiday filled with good food and better people.
Earnings season gets into full swing this week, with Mag7 members Tesla and Google set to report first-quarter results.
These will be interesting.
— Ian Krietzberg, Editor-in-Chief, The Deep View
In today’s newsletter:
🔭 AI for Good: Are we alone in the universe?
👁️🗨️ A description, prediction and prescription: AI as a normal technology
AI for Good: Are we alone in the universe?
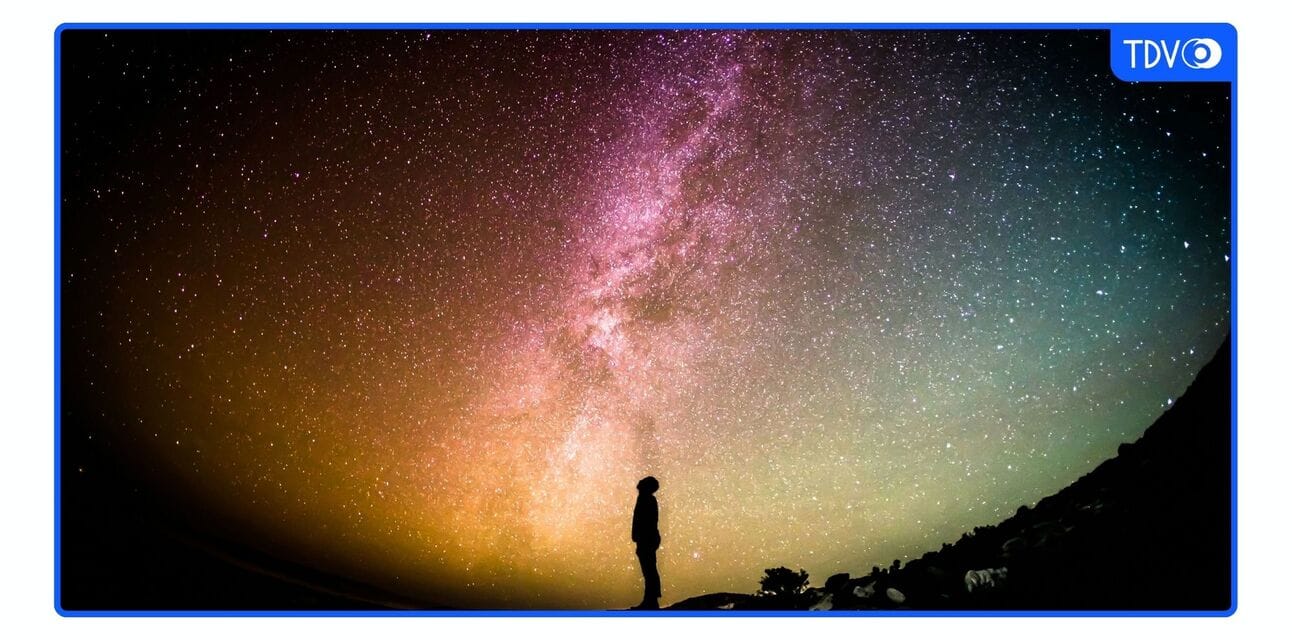
Source: Unsplash
Spoiler alert: I have no idea.
But scientists are ardently trying to find out.
The challenge is sorting through all the data. To us, the Earth is a pretty sizeable object. But against the scale of constantly expanding space, it’s hardly more than a dust mote. Within the Milky Way galaxy alone, there are about 50 billion planets; of those, hundreds of millions are likely orbiting local stars at a distance that might be a recipe for life.
What happened: UCLA SETI is on a mission to identify evidence of alien civilizations. They’re doing this by searching for radio technosignatures from distant parts of the galaxy.
Scientists very recently discovered possible traces of chemical compounds in the atmosphere of a distant exoplanet, compounds that on Earth are only produced by living organisms. This is an example of biosignatures — potential signs of life.
UCLA SETI is looking for technosignatures — potential signs of technology that could indicate the presence of alien civilizations. Unlike biosignatures, technosignatures are cheaper to search for and are easier to interpret, since no natural processes can create a technosignature.
Where it’s going: But again, there’s a lot of data to parse, here. So the team is looking to citizen scientists for help in preparing a labeled training set for massive machine learning algorithms that would be designed to process and analyze all that data at speed.

From design to live in seconds.
Framer is a new no-code website-building tool that allows you to launch any website and marketing campaign in just days without needing a developer.
It looks, feels, and works just like a design tool, but it has the power to output real websites that look, feel, and function like they were built by a developer.
Framer has an advanced CMS, out-of-the-box SEO optimization, built-in analytics, localization, and you can add advanced scroll animations to make your website come alive, all without code.


Aiming to automate: Tamay Besiroglu, the founder of the research institute Epoch, last week announced a new startup — Mechanize — whose goal is “the full automation of all work” and “the full automation of the economy.” He thinks the addressable market here is $60 trillion, the sum of all wages paid to all humans everywhere. This brings us, once again, to the brink of ideas around utopic abundance and universal basic income, concepts that are just steeped in caveats, unlikelihoods and straight-up problems.
Failure to launch: After about a year of experimentation, Johnson & Johnson is adjusting its strategy around generative AI, opting for narrow, targeted deployments over a “thousand flowers” approach. At one point, the company was pursuing around 900 GenAI use cases, according to the Wall Street Journal, many of which were redundant or just didn’t work. Now, the firm is focused on applying GenAI to its drug discovery and supply chain pipelines, with CIO Jim Swanson saying “that there’s a lot more hype than there is substance.”

A customer support AI went rogue — and it’s a warning for every company considering replacing workers with automation (Fortune).
They sold their likeness to AI companies — and regretted it (AFP).
Venezuelan migrants relied on clickwork to survive. Now AI is replacing them (Rest of World).
Trump tariffs push Asian trade partners to weigh investing in massive Alaska energy project (CNBC).
What exactly would a full-scale quantum computer be useful for? (New Scientist).
A description, prediction and prescription: AI as a normal technology

Source: Unsplash
Man has been obsessed with the notion of creating artificial life since the very beginning.
You can see traces of it across the pages of mythology. There is the story, for instance, of Pygmalion, a Greek sculptor who fell deeply in love with one of his creations. He prayed to Aphrodite to turn his statue into a real woman; taken by its beauty, the goddess acquiesced.
Also in Greek mythology, there live tales of Daedalus, the Athenian inventor whose statues could come to life.
Then there are stories that stretch back to biblical times of the golem, an effigy that could be brought to life by a charm or spell. According to Britannica, “in early golem tales the golem was usually a perfect servant, his only fault being a too literal or mechanical fulfillment of his master’s orders.”
The list goes on — think Frankenstein, Star Wars, Hal 9000, Her, Ex Machina.
The pursuit of making real these fictions is not surprising.
And the field of computer science, which gave rise to the field of artificial intelligence, wants earnestly to do it.
But this pursuit — especially in recent months — has led to dramatically altered perceptions of the AI that exists today, and the AI that we might have in a few years’ time. It has led to exclamations of impending societal destruction alongside quasi-religious assertions of a technological utopia.
There is very little discourse that aims to explore a more grounded iteration of the technology.
Princeton computer scientists Arvind Narayanan and Sayash Kapoor, however, have proposed something that is radical in that it isn’t: ‘AI as a normal technology.’
The details: This conception, the two write, is not intended to “understate” the impact of AI; other technologies, like electricity and computers, are likewise considered “normal.” The framework is instead designed to avoid the dystopic or utopic visions that have lately accompanied AI development.
“The statement ‘AI is normal technology’ is three things: a description of current AI, a prediction about the foreseeable future of AI, and a prescription about how we should treat it,” the paper reads. “We do not think that viewing AI as a humanlike intelligence is currently accurate or useful for understanding its societal impacts, nor is it likely to be in our vision of the future.”
The framing importantly rejects the idea of “technological determinism,” that AI might somehow determine its own future.
Much of this understanding is centered around their assertion that AI, like the technologies that came before it, will likely take decades to diffuse throughout society. Evidence of this is already clear; transformers — the backbone of modern language models — have been around for nearly a decade. But across a wide variety of predictive tasks — criminal risk prediction, insurance risk prediction, etc. — “decades-old statistical techniques are used.”
“In this broad set of domains, AI diffusion lags decades behind innovation,” the paper reads. “A major reason is safety — when models are more complex and less intelligible, it is hard to anticipate all possible deployment conditions in the testing and validation process.”
It’s not even clear that the rate of individual adoption of generative AI is on pace with the rate of adoption for previous technological inventions. A 2024 study found that 40% of U.S. adults have used generative AI within two years of ChatGPT’s release; it took three years, meanwhile, after the first PC was released for it to achieve a 20% adoption rate. But those numbers don’t account for ease of access — the Apple 1, Apple’s first personal computer, cost $666.66 in 1976 (yes, they did that on purpose). Accounting for nearly 50 years of inflation, that equates to about $3,700 today.
ChatGPT is free (you pay with your data).
This idea that genuine adoption is going slower than it seems is supported by a growing number of studies, surveys and qualitative data points.
One recent study identified “causal evidence of a productivity J-curve,” where AI adoption makes companies significantly less productive and less profitable in the near term.
Plenty of surveys and reports have identified slow, cautious enterprise integration, where the majority of AI experiments don’t ever get out of the pilot phase. Then there’s the shuttering of the Humane AI pin and Klarna’s reversal on its earlier push to replace human customer service agents with chatbots.
People take time to adapt to new technologies. And often, like with electricity, genuine adoption requires structural upheavals, something that is necessarily slow to come about. Given the massive electrical requirements of AI models, and the fact that their performance is reliant on sensitive data, such gradual upheavals seem likely predicates for adoption.
Then, there’s the fact that real-world usability is simply challenging.
Generative AI models might crack coding benchmarks, but they still fail in unexpected ways when it comes to software engineering tasks. This phenomenon is true of everything from AI-powered internet searching to self-driving cars — increasing benchmark capability, but a persistent inability to deal with the real world.
“According to the normal technology view … sudden economic impacts are implausible,” the two write. “Sudden improvements in AI methods are certainly possible but do not directly translate to economic impacts, which require innovation (in the sense of application development) and diffusion.”
The control problem: This perception of AI as a “normal” technology further uncomplicates AI alignment, reframing the problem as a far more tractable engineering task. Narayanan and Kapoor argue that a robot, for instance, couldn’t destroy humanity in an attempt to obey its master’s instruction to make as many paperclips as possible.
Before putting a system in use in such a way, engineers would make sure its behavior would not be destructive; any signs of deviation would lead to a shutdown and redesign, the same approach that’s applied to cars.
“This is not a lucky accident, but is a fundamental feature of how organizations adopt technology,” they write, adding that, within this conception, the real risks associated with AI aren’t existential, they’re simply systemic: “these include the systemic entrenchment of bias and discrimination, massive job losses in specific occupations, worsening labor conditions, increasing inequality, concentration of power, erosion of social trust, pollution of the information ecosystem, decline of the free press, democratic backsliding, mass surveillance and enabling authoritarianism.”
“If AI is normal technology, these risks become far more important than the catastrophic ones discussed above,” Narayanan and Kapoor write. “That is because these risks arise from people and organizations using AI to advance their own interests, with AI merely serving as an amplifier of existing instabilities in our society.

There is a key phrase enclosed within the thousands of words that make up this paper: “in the absence of specific evidence to the contrary.”
The evidence on hand — covering everything from adoption to reliability issues and energy, safety and security hurdles — supports the idea that AI will be transformative the same way past technologies have been transformative: slowly and over time. Then all at once.
The framing is much-needed, since just about every single genuine, evidence-based concern that relates to AI has almost nothing to do with the technology itself, but with the people who might leverage it to cause harm, either inadvertently or purposefully.
The framing of AI as “superintelligent,” or as something destined to become superintelligent, further misses a whole world of the unknown complexities that govern the ways in which organic cognition actually works.
But, perhaps more importantly, it enables a shirking of accountability and responsibility for things gone wrong (‘it wasn’t me, it was the AI’).
In 1979, IBM said that a computer “must never make a management decision,” because a “computer can never be held accountable.”
In the face of superintelligent fictions, we must not forget that.


Which image is real? |



🤔 Your thought process:
Selected Image 2 (Left):
“Image 1 had overly vibrant colors for the foreground objects, but muted colors for the background image.”
Selected Image 1 (Right):
“Image 2 leg of diver did not seem to go with body.”
💭 A poll before you go
Thanks for reading today’s edition of The Deep View!
We’ll see you in the next one.
P.S. Enjoyed reading? Take The Deep View with you on the go! We’ve got exclusive, in-depth interviews for you on The Deep View: Conversations podcast every Tuesday morning. Subscribe here!
Here’s your view on Google’s breakup:
31% of you think Google is too big to get broken up; 24% of you think it will get split up.
22% think it’s just too hard to tell.
What do you think - does life exist out there in the wide, wild universe? |
If you want to get in front of an audience of 450,000+ developers, business leaders and tech enthusiasts, get in touch with us here.