- The Deep View
- Posts
- ⚙️ Contextual AI: Building the next generation of RAG
⚙️ Contextual AI: Building the next generation of RAG
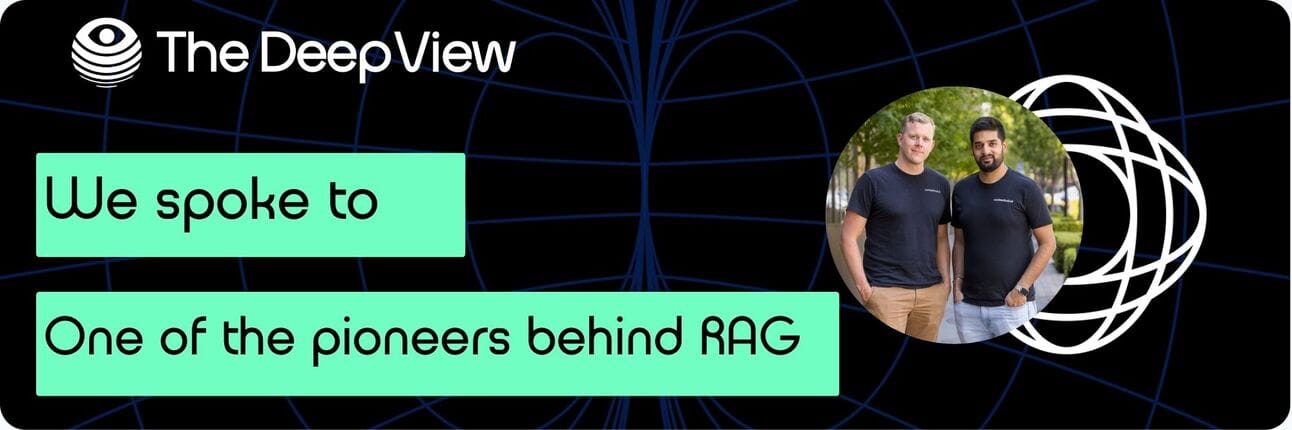
Good morning. I recently met up with Douwe Kiela, who co-authored the original paper on RAG (retrieval-augmented generation).
He’s working on Rag 2.0, a better iteration of the original RAG protocol.
— Ian Krietzberg, Editor-in-Chief, The Deep View
In today’s newsletter:
AI for Good: Malaria mitigation

Source: Zzapp
Bed nets — loaded up with insecticides — have been the main method of malaria protection and mitigation since the 1990s. Still, the World Health Organization tracks hundreds of millions of malaria cases, and hundreds of thousands of deaths each year.
Zzapp is leveraging artificial intelligence to find a better solution.
The details: The company developed an AI-powered software system — that connects to a mobile app — that analyzes satellite images and topographical maps to track mosquito populations and identify malaria transmission hotspots.
The system then sends optimized, location-based mitigation strategies to workers in the field, resulting in quick action.
In an eight-month operation Zzapp conducted on the island of São Tomé, Zzapp reduced the mosquito population by 75% and malaria cases by 52%.
The company says that its system is twice as cost-effective as bed nets.

Accurate, Explainable, and Relevant GenAI Results
Vector search alone doesn’t get precise GenAI results. Combine knowledge graphs and RAG into GraphRAG for accuracy, explainability, and relevance.
Read this blog for a walkthrough of how to:
Work with GraphRAG
Build a knowledge graph
Take next steps to achieve better GenAI
UN releases AI governance report

Source: Unsplash
The details behind the notion of AI governance have become a subject of fierce debate. The question of how we regulate the technology in a way that gives us the good, without the bad, doesn’t really have a clear, one-size-fits-all answer.
It is this, perhaps, that has led to the regulatory inconsistencies and uncertainties that are cyurrently on display across the world.
But it is a question that some are aiming to solve.
What happened: The United Nations recently published a lengthy report that explores the idea of governing AI, specifically for humanity.
According to the report, without governance, “AI’s opportunities may not manifest or be distributed equitably. Widening digital divides could limit the benefts of AI to a handful of States, companies and individuals.”
The report said that there is currently a “governance deficit” in AI; despite the regular discussion of ethical princples in the space, accountability remains null and void, compliance remains voluntary and systems remain opaque and unexplainable.
In line with what Dr. Gary Marcus has repeatedly called for, the report outlined the need for global governance.
“National governments and regional organizations will be crucial, but the very nature of the technology itself — transboundary in structure and application -– necessitates a global approach.”
You can read the full report here.

Mark Your Calendars: ZapConnect is Just 2 Days Away!
On September 26, dive into the future of automation and AI at ZapConnect’s free virtual conference. This is your chance to connect with industry pioneers, discover cutting-edge workflows, and elevate your business to new heights.


Join us for an inside look at how Ada and OpenAI build trust in enterprise AI adoption for customer service, starting with minimizing risks such as hallucinations. Viewers will also be able to participate in a Q&A.
September 26, 1PM ET/10AM PT. Sign up for the webinar today! *

X will let people you’ve blocked see your posts (The Verge).
Exclusive: Meta's AI chatbot to start speaking in the voices of Judi Dench, John Cena, others, source says (Reuters).
Anthropic has floated a $40 billion valuation in funding talks (The Information).
Vietnam, US firms sign MoUs on energy, AI, data centre, Vietnam govt says (Reuters).
Microsoft’s GitHub gives clients option to keep sensitive code in EU only (CNBC).
If you want to get in front of an audience of 200,000+ developers, business leaders and tech enthusiasts, get in touch with us here.


Study: Covert racism in LLMs

Source: Unsplash
One of the most prominent limitations of large language models (LLMs) involves bias, which, taking a number of different forms, and has many implications for the usefulness, reliability, efficacy and fairness of models and systems based on this architecture.
A recent study published in Nature found that this bias includes something called “covert racism.”
The details: The researchers investigated covert racism through dialect prejudice, focusing on AAE (African American English). This investigation, they said, is different than others, because the race of the speakers is never overtly stated.
The researchers observed a “discrepancy between what language models overtly say about African Americans and what they covertly associate with them as revealed by their dialect prejudice.”
“This discrepancy is particularly pronounced for language models trained with human feedback (HF), such as GPT-4: our results indicate that HF training obscures the racism on the surface, but the racial stereotypes remain unaffected on a deeper level.”
Why it matters: In instances where systems based on LLM architecture are being used for decision-making (such as employment, criminality or legal proceedings and healthcare), such under-the-surface biases could have massively harmful implications for a lot of people.
Contextual AI: Building the next generation of RAG

Source: Contextual AI
Large language models (LLMs) are constrained by their training data. Retrieval-augmented generation (RAG) was proposed as a solution; in short, RAG refers to a technique that combines an LLM with an external data source, improving the model’s performance, explainability and transparency through access to targeted, up-to-date information.
I spoke with Douwe Kiela — the CEO of Contextual AI, an adjunct professor at Stanford and one of the co-authors of the original RAG research paper — about the next generation of RAG that he’s currently building.
Welcome to RAG 2.0: Part of Kiela’s idea of RAG 2.0 has to do with the fact that, in its current form, RAG isn’t really being used properly. He said that the idea of the original paper was that you can “train the retriever and the generator at the same time.”
But a lot of people are using the “easy, naive” version of RAG, where those two components are trained separately. He referred to this as “Frankenstein’s RAG.”
RAG 2.0 is, instead, a continuation of the trend highlighted in the original paper, with those two parts working together.
“An analogy I always like using is of a brain … you have these two halves of the brain, the retriever and the generator. You know that you want them to work together, so you're going to use the brain in a RAG setting, but in the Frankenstein paradigm, they're just completely separate. So it's kind of miraculous that it even works,” he said. “In the RAG 2.0 paradigm, we're going to make these two halves of the brain literally grow up together, so that they're very aware of each other, that they really work in lockstep.”
Last month, Contextual AI announced that it had closed an $80 million Series A funding round led by Greycroft, with participation from a list of investors that included Bezos Expeditions and NVentures (NVIDIA’s venture capital arm).
Kiela told me that the company was “born from this frustration that we could see in enterprises everywhere after ChatGPT came out,” that for all the genAI hype, the technology was — and remains — fundamentally limited by hallucination and inexplainabilty.
His focus with Contextual is simply to provide a reliable generative AI solution for the enterprise. Nothing more.
No AGI here: Conspicuously lacking from Contextual’s website is any mention of artificial general intelligence (AGI), which refers to a hypothetical AI that would be on par with human intelligence. OpenAI, for example, is openly working to build AGI. Some researchers remain skeptical that it will ever be possible.
But Contextual doesn’t deal in AGI, and Kiela said that this “deliberate choice” represents one of the main ways in which Contextual differs from the competition: it’s focused only on enterprise problems.
“AGI, fundamentally — we think — is a consumer product. And the reason for that is that with consumers, you don't really know what they want, so you need to be able to do everything,” he said. “But in an enterprise, you almost always know exactly what you want from the system. So it's a much more constrained problem in a good way … you can solve it much better through specialization.”
The other point of difference is the company’s focus on systems over models; models, Kiela said, are important, but they only represent one fraction of an ecosystem that ought to include other things — guardrails and RAG, for instance. “It’s not just a language model, it’s how you contextualize the model.”
The “system doesn't need to know about quantum mechanics and Shakespeare when it's solving a particular problem in an enterprise,” he said. “It just has to do the one thing really, really well, and that's what we do.”


Which image is real? |



🤔 Your thought process:
Selected Image 2 (Left):
“The reflective highlights looked more natural, the other image looked like it was lit by two light sources plus the orange hue looked too brilliant compared to how the planes typically look in the air.”
Selected Image 1 (Right):
“Image 1 was having more details and looking rough, indicating to be real.”
💭 A poll before you go
Thanks for reading today’s edition of The Deep View!
We’ll see you in the next one.
Here’s your view on LLMs and math:
34% of you said LLMs are okay with math problems, depending on the complexity of the problem. 19% said they work terribly and 13% said they work great.
22% of you do your math with a paper and a pencil, no LLMs needed.
It’s terrible:
“Given that generative AI built with LLMs can not fathom the meaning of the prompts, it is really not a surprise that they fail miserably at mathematics. We are still a long way from AGI. Until we understand what understanding truly requires, what is necessary and sufficient to cause meaning to arise, AI is stumbling around in a lightless room, hoping to find a light switch.”
Do you use RAG? |